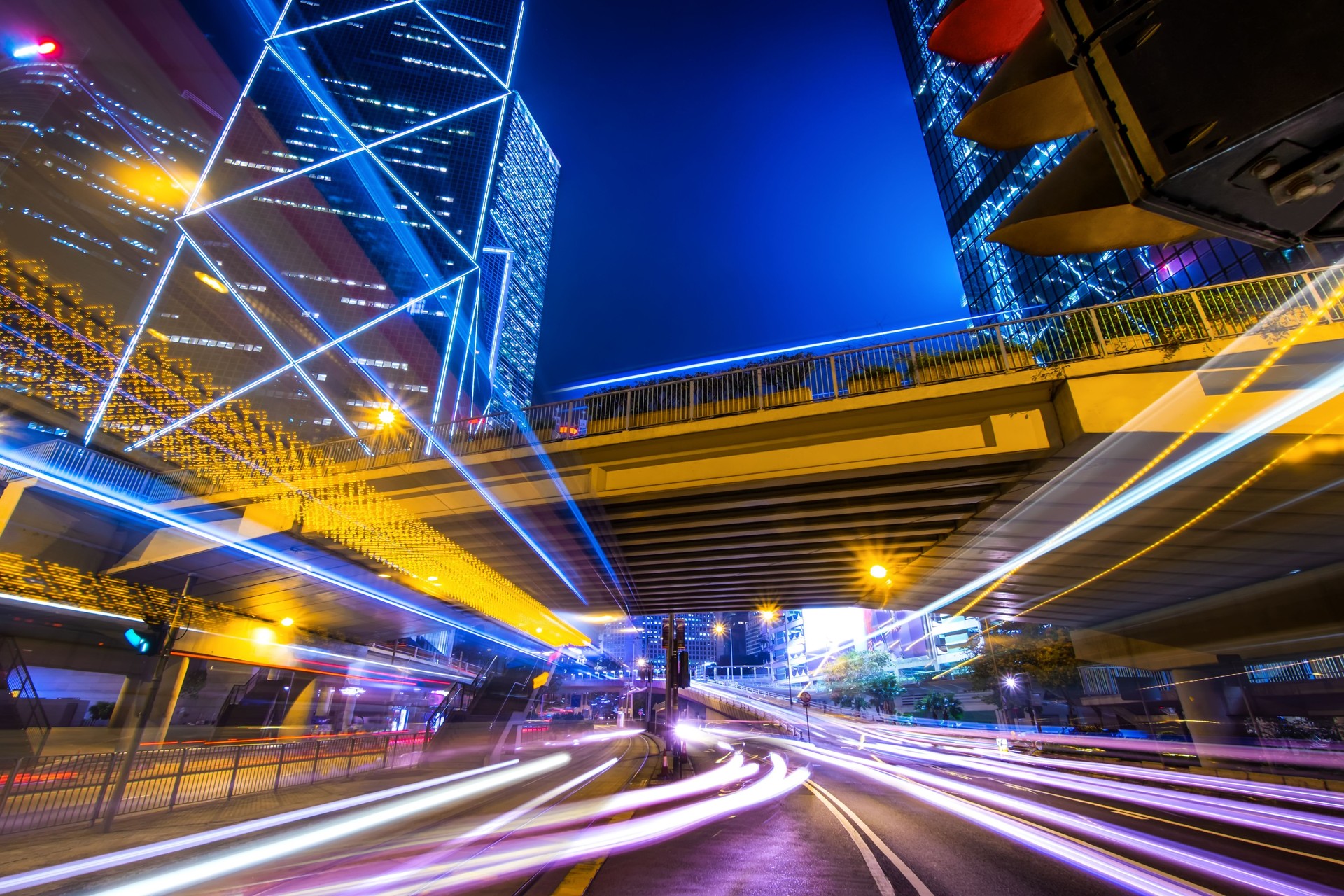
Transforming road maintenance with AI: smarter, safer and more efficient infrastructure management
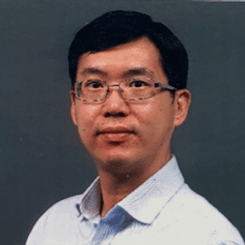
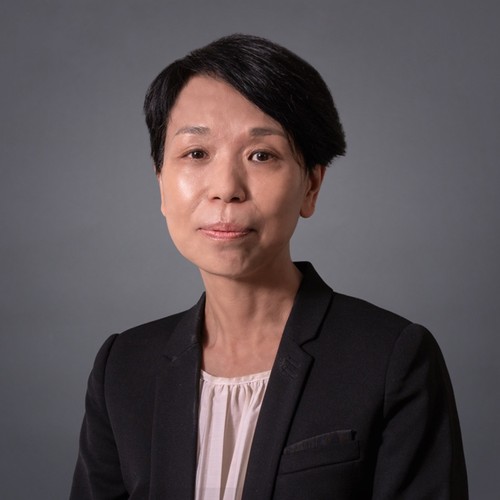
Maintaining safe, reliable road networks is critical for economic growth and public safety. Yet, as urban infrastructure ages and traffic volumes rise, traditional road inspection methods are struggling to keep pace. Manual inspections, which rely on engineers visually assessing road conditions, are time-consuming, resource-intensive and prone to inconsistencies.
In Hong Kong, where the Highways Department (HyD) oversees approximately 2,200 kilometers of public roads, these challenges are amplified. Each district typically requires about three to four months to complete an inspection cycle, and heavy traffic conditions make manual inspections difficult. This often leads to delays in defect detection and subsequent repairs. As a result, this reactive approach not only increases maintenance costs but also poses safety risks for road users.
The cost of inefficient road inspections
Traditional road maintenance relies on periodic visual assessments, allowing cracks, and discolored road markings to go unnoticed for long periods. Manual inspections require extensive personnel deployment, making them costly and time-consuming. In dense urban areas like Hong Kong, heavy traffic further complicates access and delays repairs.
The reliability of manual inspections varies due to factors like inspector expertise, environmental conditions, worker safety and time constraints.
These limitations result in broader challenges for maintenance systems:
- Escalating maintenance costs: Minor defects become major over time, leading to more extensive and expensive repairs.
- Compromised road safety: Poor surface conditions and unclear markings increase the risk of accidents, especially in areas with heavy traffic.
- Strained resources: Limited manpower and budgets make it difficult to prioritize repairs without timely, reliable data.
Harnessing data for smarter cities
To address growing infrastructure challenges, many cities are adopting data-driven approaches to road maintenance. By using technology like sensors, GPS and AI-based analysis, governments can monitor road conditions more frequently and prioritize repairs.
Chicago, for instance, developed a system that analyzes service requests submitted through its 311 non-emergency system and other data to identify pothole hotspots. This helps crews proactively target high-risk areas and improve repair efficiency.
Beyond road maintenance, cities are also deploying similar approaches in other areas. Seoul, for example, uses a citywide internet of things (IoT) network with connected sensors to monitor traffic, infrastructure and environmental conditions in real time, helping authorities respond quickly and plan more effectively.
Below images: green = intact road marking, red = discolored road marking (with shared area of paint loss), blue = cracks identification
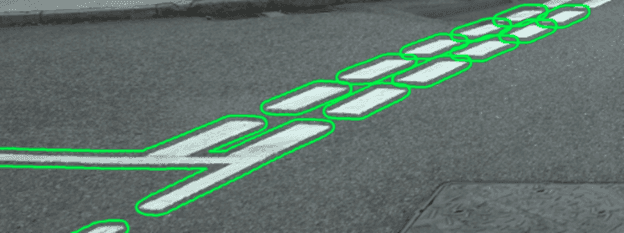
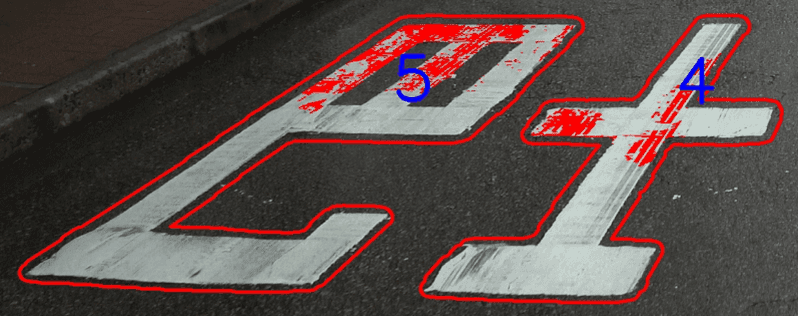
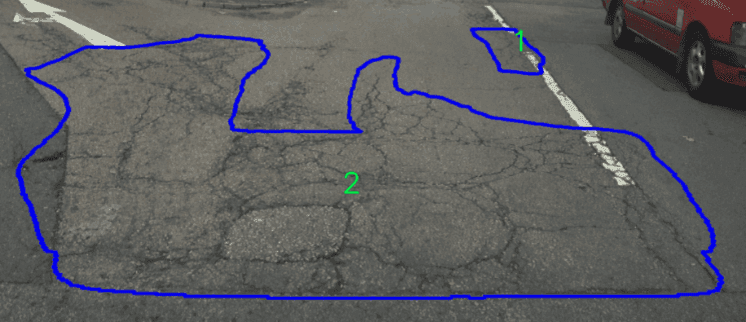
A smarter solution: the Road Defect Detection System (RDDS)
Identifying a unique opportunity to innovate with emerging technology, the HyD engaged AECOM to develop the Road Defect Detection System (RDDS), an AI-powered solution that integrates high-resolution imaging, geospatial analysis and machine learning for precise defects detection.
The RDDS consists of three core components:
- Road Imaging and Capturing Service (RICS): A vehicle-mounted system that captures geo-tagged, high-resolution images along the road network.
- AI-powered defect detection: Machine learning algorithms analyze images to identify and classify defects, providing detailed insights on size, severity and exact location.
- Web-based GIS platform: A digital interface where engineers can search defects, prioritize repairs and allocate maintenance resources efficiently. Additionally, it enables engineers to report both false positives or false negatives.
Modernizing road maintenance with AI-powered precision
The RDDS standardizes and automates defect detection while continuously improving accuracy. In its initial deployment, it achieved a 90% accuracy in identifying road defects, eliminating the subjectivity of traditional inspection methods and enabling more data-driven maintenance planning.
For road markings, the RDDS uses precision-based measurement, categorizing discolored road markings by paint loss and flagging those exceeding the pre-defined threshold. For road cracks, advanced pattern recognition classifies defects into area-based or linear patterns, allowing for better prioritization and remediation.
One standout feature of the RDDS is its ability to let users report incorrectly detected defects. Each time an inspector verifies or flags a defect as incorrect, the system records the result along with related data. This information is then used to improve the AI model, increasing its accuracy over time. Through this adaptive learning process, the RDDS reduces false positives and stays responsive to real-world conditions, enhancing overall reliability. Additionally, the RDDS significantly shortens the road inspection cycle from three months to just three weeks and contributes to better overall road conditions.
Below image: Road Defect Detection System (RDDS) portal
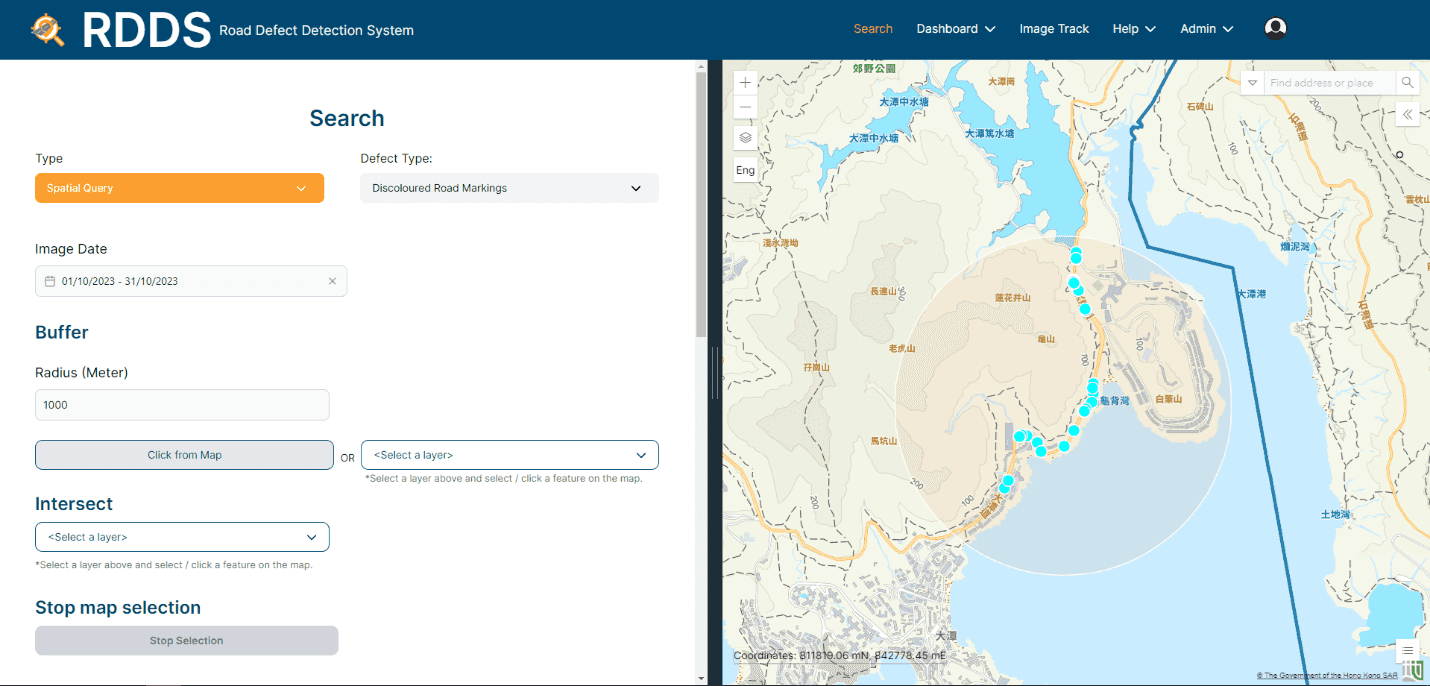
Technical considerations for future enhancements
As the RDDS continues to evolve, it offers significant promise for improving road maintenance. However, several technical and operational considerations must be addressed to fully realize its potential. Ongoing enhancements aim to resolve current limitations while expanding the system’s capabilities.
Current challenges and key considerations include:
- Computational and storage demands: The RDDS generates a large volume of high-resolution images, requiring significant processing power and storage capacity. Supporting efficient real-time data processing calls for further investment in hardware, cloud infrastructure and AI resources.
- Limited detection scope: Currently, the RDDS focuses on detecting discolored road markings and road cracks. Work is underway to expand its capabilities to include other hazards such as faded signage, vegetation encroachment, potholes and surface deformations that impact road safety.
- AI accuracy and verification: Although the models are highly effective, they are not infallible. Instances of false positives and false negatives highlight the continued need for human oversight to maintain accuracy and reliability.
- Subsurface defect limitations: Because the RDDS relies on visual imaging, it is limited in its ability to detect subsurface issues, such as structural weaknesses that are not visible on the surface. Complementary technologies, such as ground-penetrating radar and acoustic sensors, may be incorporated to support more comprehensive assessments.
- Integration with existing asset management systems: The volume and complexity of data produced by the RDDS may not integrate easily with legacy maintenance platforms or enterprise asset management systems. Achieving interoperability will require system upgrades, streamlined data handling and improved compatibility.
- Data standardization: Efforts to standardize defect classifications are important to support benchmarking across jurisdictions and to promote consistent, effective infrastructure management.
- Privacy considerations: Since the RDDS captures imagery from public roads, anonymization protocols play a key role in building public trust and supporting responsible use of AI-driven inspection technologies.
Looking ahead, predictive analytics will play a critical role in enabling a shift from reactive to proactive maintenance planning. By combining RDDS data with historical maintenance records, traffic information and environmental conditions, authorities will be better equipped to anticipate deterioration, reduce long-term repair costs and make smarter infrastructure decisions. The system also has the potential to evolve into a digital twin of the road network, offering a more dynamic and data-driven approach to road management.
In the future, the RDDS may be integrated with robotics and drones to access hard-to-reach areas for inspection. Additionally, combining the IoT and crowdsourcing through ride-hailing vehicles can enable the collection of real-time updates on road conditions. Leveraging existing ride-hailing fleets reduces the need for dedicated inspection vehicles. While this approach may introduce additional integration challenges, continued innovation is expected to support these advancements and further extend the system’s reach and impact.
Delivering safer roads through AI-based predictive maintenance
As urbanization accelerates, data-driven solutions are becoming essential to keeping roads safe and reliable. The future of road maintenance relies on collaboration, innovation and investment, with AI-powered technologies like the RDDS playing a key role in strengthening infrastructure and improving long-term resilience.
By leveraging data and automation, road management can move beyond routine maintenance to a more predictive and proactive approach. As AI accuracy, interoperability and predictive modeling continue to improve, cities can enhance road safety, increase efficiency and build more resilient infrastructure for the future.